
Unknown Psychoactive Substances Screening
(Photo: HTX)
HTX has developed an Artificial Intelligence tool to classify Novel Psychoactive Substances (NPS), even those which have not yet been recorded. NPS are synthetic substances which seek to emulate existing ones such as ecstasy, cathinone, and heroin, posing a global issue not just by their harmful effects but also by their ever-increasing diversity. Just in 2021 to 2022 alone, 76 new variants were found.1 Gas Chromatography Mass Spectrometry (GC-MS) is a common tool used by drug enforcement agencies to match spectra of seized substances against a library recorded from known substances. However, GC-MS measurements can yield different results for derivatives stemming from the same family of chemicals, making it hard to identify seized substances which are unrecorded NPS (Figure 1). This is exacerbated by the increasing numbers of NPS variants, causing a lag in updating an incomplete library with newly identified drugs.
Figure 1. Both NPSes are synthetic cannabinoids with a slight molecular variation but has varied GC-MS measurement outcomes. (Photo: HTX)
The HTX AI classifier is built by training a machine learning model with open-sourced GC-MS data (approximately 700 from NPS and 200 from non-NPS compounds). The HTX AI classifier takes in background subtracted and normalised GC-MS data and outputs the predicted drug class that the unknown NPS belongs to. The tool predicts if the measured substance belongs to one of six different NPS drug classes and their analogues, namely, cathinones, cannabinoids, phenethylamines, piperazines, tryptamines, fentanyls or of non-related compounds (Figure 2).
Figure 2. The HTX AI classifier for NPS identifies a cathinone. (Photo: HTX)
To build this classifier, we had to overcome the issue of a relatively small training dataset size. This was done using data augmentation mimicking variations in actual GC-MS measurements and was implemented by randomly scaling the fragment abundances by ±10% of their values and renormalising them. This increases the dataset size by 10x and improves the performance of the trained model. Benchmarked against a simple spectra match factor method, as employed in NIST Mass Spectral Search Program (MS Search) on a simulated incomplete database, our HTX AI Classifier shows a two-fold increase in accuracy (Figure 3). Preliminary validation of our model on actual lab measurements indicates F1 scores as high as 90%. For further technical details, we refer the reader to our published article in Forensics Chemistry.2
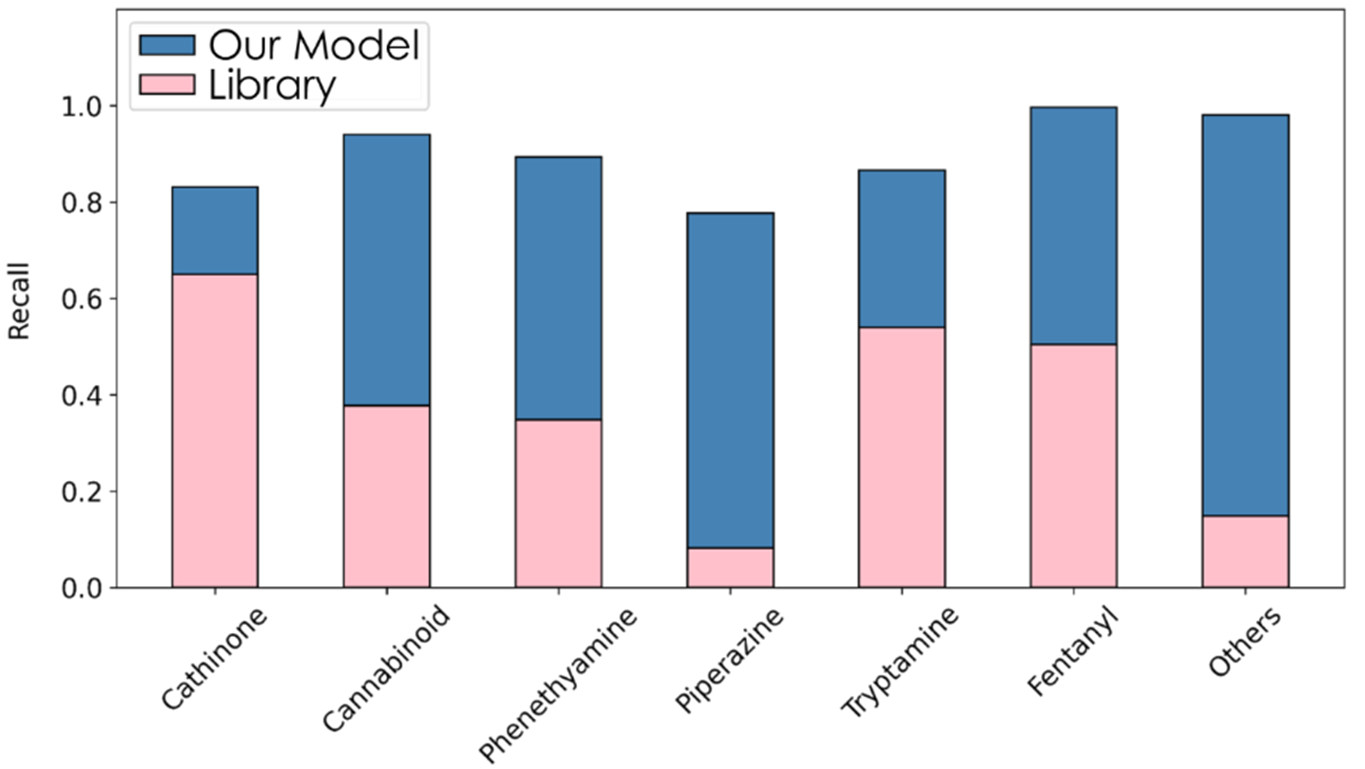
Figure 3. Benchmark scores of library matching method versus HTX AI Classifier Model (Reproduced from journal article)2
We are currently working on expanding the diversity of drug classes predicted for and exploring an end-to-end integration with existing GC-MS equipment. With further development, our work can potentially increase the speed with which unknown NPS substances are identified at our borders and aid decision making processes.
Coming Next
- We intend to expand the diversity of NPS classes to be predicted for, with further validation using lab measured samples.
- If the model continues to perform well on our own lab data, we look forward towards integrating it with current instruments to enable an end-to-end process from initial measurement to engaging the AI model.
Interested to find out more or discuss further?
Do connect with us: HTX_TechX@htx.gov.sg
References
- UNODC, Current NPS Threats, Volume V, Oct 2022.
- S.L. Wong et al., Forensics Chemistry 34, 100499 (2023).
Related Links
- Screening unknown novel psychoactive substances using GC–MS based machine learning, S.L. Wong, L.T. Ng, J. Tan, J. Pan, Forensics Chemistry 34, 100499 (2023).
- Enlisting Artificial Intelligence in the fight against Novel Psychoactive Substances
- TechXplore: Exponentially Impacting Singapore’s Safety and Security (Singapore Pavilion)